Hosted by
Occupant behavior and energy consumption in dwellings: An analysis of behavioral models and actual energy consumption in the Dutch housing stock
Synopsis
Much is known about the increasing levels of energy consumption and environmental decay caused by the built environment. Also, more and more attention is shown to the energy consumption of dwellings, from the early design stage until the occupants start living in them. The increasing complexity of building technologies, the occupants’ preferences, and their needs and demands make it difficult to achieve the aimed energy consumption levels. The goal of reducing the energy consumption of dwellings and understanding the share of occupant behavior in it form the context of this research.
Several studies have demonstrated the ‘energy performance gap’ between the calculated and the actual energy consumption levels of buildings, and have explored the reasons for it. The energy performance gap is either caused by calculation drawbacks, uncertainties of modeling weather conditions, construction defects regarding air tightness and insulation levels, or by occupant behavior. This research focuses on the last aspect, i.e. analyzing the relationship between occupant behavior and energy consumption in dwellings, understanding the determinants of energy consumption, and finding occupants’ behavioral patterns.
There are several dimensions of occupant behavior and energy consumption of dwellings: dwelling characteristics including the energy and indoor comfort management systems, building envelope, lighting and appliances; occupant characteristics including the social, educational and economical; and actual behavior, including the control of heating, ventilation and lighting of spaces, and appliance use, hot water use, washing, bathing, and cleaning. Attempting to understand this complexity asks for a methodology that covers both quantitative and qualitative methods; and both cross-sectional and longitudinal data collection, working interdisciplinary among the domains of design for sustainability, environmental psychology, and building and design informatics.
The main question that this thesis deals with is: How much does the occupant behavior influence the energy consumption of dwellings in the Netherlands, and how could we identify the determinants of consumption, as well as the behavioral patterns and profiles?
In order to research this question, the following questions are formulated:
- I What is the sensitivity of a dwelling’s heating energy consumption to occupant behavior? (Chapter 3)
- What are the existing models developed for the occupant behavior and energy performance relationship? and how different are the results of these models in terms of calculating the influence of occupant behavior on energy performance?
- How can behavior be modelled in order to assess the robustness of the energy performance in dwellings to occupant behavior?
- What is the weight of each behavioral aspect in terms of its influence on energy consumption?
- II What is the influence of lighting and appliance use on the total electricity consumption in dwellings? (Chapter 4)
- What are the main direct and indirect determinants of electricity consumption? (Direct determinant: such as number of appliances and duration of appliance use …; Indirect determinant: such as household size, dwelling size, dwelling type …)
- How much of the variance in electricity consumption in dwellings can be explained by direct and indirect determinants?
- III What are the behavioral patterns and profiles of energy consumption?
- What are the behavioral patterns of thermostat control? How do they relate to the household characteristics, revealing behavioral profiles? (Chapter 5)
- What are the behavioral patterns of electricity consumption? How do they relate to the household characteristics, revealing behavioral profiles? (Chapter 6)
In this thesis, occupant behavior is modeled in different chapters using sensitivity, correlation, regression, repeated measures, and cluster and factor analyses, based on data on dwelling and household characteristics, actual behavior, and energy use. The structure of the thesis is based on the kind of energy use: heating energy and electricity for appliance and lighting. First, a sensitivity analysis for occupant behavior and heating energy consumption is conducted. Afterwards, determinants of occupant behavior in relation to heating energy consumption is explored through existing research. Determinants of electricity consumption for lighting and appliances are analyzed using correlation and multiple regression methods. In-depth analyses of behavioral patterns regarding heating energy are realized by repeated measures and cluster analyses, and electricity consumption by factor analysis. The research combined deductive and inductive methodologies. In this thesis, the deductive method is defined to operate on the macro level, using cross-sectional data on the dwelling and its systems, and include population data collected with one-time questionnaires and energy consumption characteristics based on yearly bills. The inductive method operates bottom up, applying monitoring and other longitudinal data collection methods and use actual data on thermostat control behavior. Research using inductive and deductive methods display a significant variance in explaining the sensitivity of energy consumption to occupant behavior.
Three datasets were used in this research. The first one is based on data collected in Wateringse Veld in The Hague, and Leidsche Rijn in Utrecht. The data was collected through a questionnaire in the autumn of 2008. The inhabitants were asked to respond to questions regarding the architectural typology, the heating and ventilation systems, the envelope properties of their dwellings, the number and use of lighting and electrical household appliances, and the energy consumption, in addition to the economical, educational and social characteristics of the household and the individual, the presence patterns in the house and in different rooms, the indoor comfort and energy management behavior patterns, habits, hobbies, and health conditions. This dataset consists of 323 dwellings.
The second dataset is comprised of 61 dwellings chosen randomly among the clients of one energy company. The household characteristics are representative for the Dutch average. Data on thermostat control behavior was collected by monitoring during March and April 2011, while a questionnaire was used for an inventory of household characteristics and behavioral attitudes, before the monitoring started.
Lastly, the WoON survey was used as a validation database for the first dataset. The WoON Database of the Dutch Ministry of Housing includes data of 4500 dwellings and is assumed to be representative for the Netherlands. This database includes a household survey, data on occupant behavior, dwelling inspections and reports on energy consumption in 4500 dwellings across the Netherlands.
In relation to the research questions, the main conclusions of this research can be summarized as follows:
Q I: Sensitivity analysis can be used as a method of evaluating the impact of occupant behavior on heating energy consumption. Heating energy consumption of a dwelling is the most sensitive to thermostat control, followed respectively by ventilation control and presence. Both heating energy consumption and the resultant indoor temperature are the most robust to radiator settings, meaning that heating energy consumption and resultant indoor temperature change minimal if the occupants change the radiator control.
Q II: Total appliance use (ownership and duration of use of appliances) is calculated based on the direct detereminants of electricity consumption. DHES (Dwelling, Household, Economic, System) characteristics of dwellings, i.e. household size, dwelling type, the number of showers, use of dryer and washing cycles are the indirect determinants, and the combined model of direct and indirect determinants explains 58% of the variance in electricity consumption.
Q III - 1: Four occupant profiles are identified for heating energy consumption: (1) no pattern, (2) one-off, (3) comforty, (4) controller. The first profile does not have significant common household characteristics, and displays no pattern of thermostat use. This profile requires detailed investigation of the household behaviors. The second profile, ‘one-off’ households pick a single set point over a period of hours (morning, day time, evening, and night time), and this is repetitive during two months. These households can be characterized with higher educated males and gadget lovers, not necessarily interested in energy saving. The third profile, ‘comforty’ households have a thermostat control of more than one set point and intervals, with high temperature preferences, in different days of the week, which is identified as a pattern during the two months. This group is composed of homeowners with a high income and larger dwellings, and are not interested in energy saving, also prefer higher temperatures. Lastly, the fourth profile, ‘controller’ households prefer one or two set point temperatures and intervals, with low temperature preferences, in different days of the week, repetitive for two months. Group four is composed of households with an energy saving agenda, mostly families and sometimes the elderly, where the parents/couples take decisions regarding energy consumption together.
Q III - 2: Behavioral factors of electricity consumption are total appliance use, the use of Information, Communication, Entertainment (ICE) devices, presence, personal hygiene and household cleaning, and energy conservation behavior. Based on these, the behavioral patterns are defined as appliance use, the use of technology / occupant presence, personal hygiene and household cleaning / occupant presence, and energy conservation. The correlations between behavioral factors, and household and dwelling characteristics reveal the behavioral profiles. These are the specific groups of users with corresponding behavioral characteristics: (1) family (couples (sometimes with a kid) with average user behavior), (2) techie (households that possess a lot of ICE devices), (3) comforty (larger households with a higher income that have a high usage of lighting and appliances, as well as heating), (4) conscious (smaller size family, elderly, lower income, higher education households who consume less, as well as owning solar panels, energy saving lamps, etc.). The behavioral patterns and the behavioral profiles are statistically significantly different from each other in relation to electricity consumption.
In relation to the main question; “how much does the occupant behavior influence the energy consumption of dwellings in the Netherlands, and how could we identify the determinants of consumption, as well as the behavioral patterns and profiles?” we could summarize the following:
This thesis has been interested in determining occupant behavior in relation to energy consumption, claiming that the buildings’ energy consumption can be validated in total, only during occupancy, when the design is tested on actual use. Referring to the lack of research, this study combined the deductive (cross-sectional, macro data, macro level statistics) and the inductive methods (longitudinal data, detailed high frequency data, performance simulation), by considering both the determinants of behavior and the actual behavior itself. We found that deductive methods are much faster in calculating and dissecting energy consumption into its factors, such as household characteristics, dwelling characteristics, behavioral aspects, etc; and inductive methods model actual behavior from bottom up experimenting and validating energy consumption levels. In addition, this research has found that the heating energy consumption of a dwelling is the most sensitive to thermostat control, followed respectively by ventilation control and presence. Both heating energy consumption and indoor resultant temperature are the most robust to radiator control. Calculating a regression model on the determinants of electricity consumption, this research has found that using the total duration of appliance use and parameters of household size, dwelling type, number of showers, use of dryer and washing cycles, and presence in rooms, 58% of the variance in electricity consumption could be explained. Introducing behavioral profiles and patterns contribute to the modeling of energy consumption and occupant behavior, this research revealed that household composition, age, income, ownership of dwelling, and education are the most important elements of behavioral profiling.
This thesis addresses occupant behavior in dwellings in the field of sustainability and building energy consumption by using interdisciplinary methodologies, i.e. by combining different modeling and data collection methods. It reveals unknown aspects of the relationship between energy consumption and occupant behavior, and reveals occupants’ behavioral patterns and profiles of energy consumption.
For the energy and indoor comfort engineering industry, the knowledge gained through this research means support for designing systems that are more effective in reducing energy consumption, in addition to influencing users towards energy efficient behaviors. For policy, building industry, and design informatics (particularly simulation based energy performance assessment and design tools), this research illustrates the benefit of considering occupant behavior in early phases of design in renovating existing housing stock and for new housing when aiming for sustainability. Furthermore, this thesis could contribute to the better design and implementation of energy control systems and products. Further research could utilize this knowledge to increase the energy efficiency of dwellings.
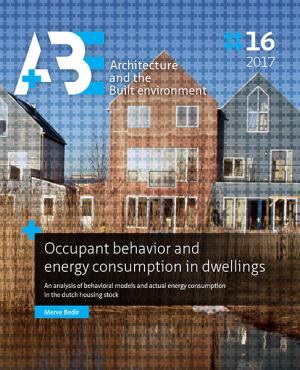