Hosted by
Predicting energy consumption and savings in the housing stock: A performance gap analysis in the Netherlands
Synopsis
The research used several large datasets, about dwellings theoretical energy performance, most of which were related to energy label certificates. All the datasets containing theoretical performance were merged with actual energy data. In addition to that, some were also enriched with socioeconomic and behaviour related data from Statistics Netherlands (CBS) or from surveys which were designed for the purpose of this research. Simple descriptive statistics were used to compare average theoretical and actual consumptions. Advanced statistical tests were used for detecting correlations, followed by several regression analyses. In a separate scenario study, the resulting averages of both theoretical and actual consumptions were extrapolated nation-wide in order to be compared with the existing policy targets.
Due to low predictive power of the variables in regression analyses, a sensitivity analysis of the theoretical gas use was performed on six assumptions made in the theoretical calculation to show how an increment in one of the assumptions affects the final theoretical gas consumption and whether this can explain the performance gap.
Last but not least, longitudinal data of the social housing dwelling stock between 2010 and 2013 was analysed, focusing on dwellings that had undergone renovation. The goal was to find out whether the theoretical reduction of consumption materialised and to what extent. A comparison of the actual reduction of different renovation measures was made in order to show what renovation practices lower the consumptions most effectively. The discrepancies between actual and theoretical heating energy consumption in Dutch dwellings.
Discrepancies between theoretical and actual gas and electricity consumption
On average, the total theoretical primary energy use seems to be in accordance with actual primary energy consumption but when looking at more detailed data, one can see that the contribution of gas to the actual primary energy is much lower than in the theoretical primary energy and that the contribution of electricity is opposite — higher in the actual than theoretical primary energy. The two effects cancel each other out so that in terms of total primary energy, the theoretical consumption seems to be well predicted. Furthermore, the analyses showed that the variation in electricity consumption is marginal across label categories. This together with the fact that most Dutch dwellings are heated with gas made us focus exclusively on gas consumption in the rest of the thesis. Whereas it is clear that theoretical electricity consumption is much lower than actual since it does not account for appliances, however, it is much less obvious why gas consumption is on average so much lower in reality than according to theoretical calculation.
Performance gap in relation to energy label
The discrepancies in gas consumption were the largest in the poorest performing dwelling, where theoretical consumption surpassed the actual almost twice, which we also referred to as overprediction. On the other hand, well performing dwellings consume roughly 20% more gas than predicted. Theoretical electricity consumption was at least twice lower than actual in all label categories, due to the fact that actual consumption takes into account electricity use of appliances and theoretical does not. Actual and theoretical electricity consumptions seemed to be rather constant with regard to the label class. Primary energy consumption is a sum of consumption of gas and electricity in MJ for each label class where the efficiency of the electricity generation and of the network was taken into account as well as the heating value of gas burning. The theoretical primary energy use is dominated by gas consumption, since electricity is a relatively small fraction of primary energy use due to exclusion of the household appliances. The relation between actual and theoretical therefore remains similar as seen in gas consumption. For poor label classes, the theoretical consumption is overpredicted by about 30% and for good label classes it is underpredicted for roughly the same percentage.
Electricity consumption does not seem to depend on the energy performance of the dwelling. Moreover, the end uses of electricity included in actual and in theoretical consumption are different to an extent that renders a comparison meaningless (as the theoretical excludes appliances). Therefore the main focus of the thesis was gas consumption, which is also the predominantly used fuel for heating homes in The Netherlands.
Performance gap in different samples
The performance gap was analysed in four different datasets of varying size. All datasets provided very comparable results regarding average actual and theoretical consumptions across label categories. A closer analyses shows that the actual gas consumption has been dropping steadily within label categories A, E, F and G from 2010 till 2012. Theoretical gas consumption remained roughly the same in these years, which means that the performance gap has increased slightly.
Moreover, it was found that the dwellings which had no renovation measures applied and remained unchanged from year 2010 till 2012 still exhibit a 3,5% decrease in gas use between 2010 and 2012, which shows that the decrease detected in the fours studied samples is not due to sampling bias. This decrease could be a consequence of a changing household composition (smaller number of people per household) or a decreased use of gas for cooking, however, both these phenomena’s occur at a pace smaller than 3,5%. Other factors which could be responsible for this decrease could be the changing calorific value of gas and/or the method for the calculation of standardized annual consumption.
Performance gap in relation to dwelling type, floor area and installation types
The analyses showed that floor area does not affect the performance gap strongly. In terms of dwelling type, semi-detached houses have the highest performance gap, followed by flats with a staircase entrance, detached houses and finally, gallery flats. The performance gap differed also in dwellings with different installation types. Dwellings with a local heater in the living room (gas stove) had the highest performance gap, followed by a combined boiler with η<83%, and then each higher efficiency boiler had a smaller performance gap. Energy reduction targets for built environment and actual reduction potential of the dwelling stock and of the individual dwelling renovation measures
Theoretical and actual achievability of the current targets
A scenario analyses was conducted in the third chapter. The baseline scenario was the scenario described in Covenant Energy Savings Housing Associations Sector’ (Convenant Energiebesparing Corporatiesector, 2008), which aims is to save 20% gas consumption by 2018 by improving the dwellings to a B label or at least by 2 label classes. The refurbishment scenario of the mentioned agreement was one of the scenarios considered. Another, more radical refurbishment scenario was renovating the whole dwelling stock to label A. The two scenarios were tested on both baseline consumptions, actual and theoretical (Figure 4). It turned out that by using theoretical gas use as baseline, the least radical scenario is enough to ensure the potentials discussed in B.1 are fulfilled. However, if actual gas consumption is used as a baseline, most of these potentials seem unrealistic (exception is the 10% potential as defined by IDEAL project). This points to the fact that analysts as well as policy makers rely on theoretical gas consumption as a basis for future consumption estimates, which ultimately leads to unrealistic reduction targets and renovation plans.
Differences between the theoretical and actual reductions in dwellings where different renovation measures were applied
Longitudinal data of dwellings energy performance was used to identify renovated dwellings and analyse their energy consumption before and after the renovation. The results showed that most of the renovations are expected to yield larger reduction than what materialises, many times the realised saving is about half of the expected. On average in all renovated dwellings, actual gas reduction is about a third lower than expected, however, there are big differences in the reductions of individual measures. Improvements in efficiency of gas boilers (space heating and hot tap water) yield the biggest energy reduction, followed by deep improvements of window quality. Improving the ventilation system yields a relatively small reduction compared to other measures, however, it is still much larger than theoretically expected. The measures achieving the most reduction are drastic improvements of window quality and an improvement of the efficiency of heating and hot tap water system (not a replacement of a local system). These are averages and the reductions for specific changes vary considerably. Measures that achieve an actual reduction higher that the theoretical seem to mostly be very modest improvements of insulation or window quality. Also notable is the underprediction of the reduction in dwellings where natural ventilation was replaced by mechanical exhaust and it is questionable whether such dwellings still have a sufficient quality of indoor air after the renovation. Causes of the differences between actual and theoretical gas consumption
Explaining variation in gas use with dwelling, household and occupant characteristics
Regression based on socioeconomic data showed that explaining the actual gas consumption or the difference between the actual and theoretical with the publicly available variables yields a relatively low R2 value (in view of existing literature these R values are not low) of 50,5% and 44,0%, respectively, meaning that 50,5% of the variance could be explained by these factors. Since our dataset contained many records, this relatively low explanatory power was thought to be due to the fact that many factor that do influence actual energy use, such as indoor temperature or presence of occupants, were not included. In the regression based on the survey data, these factors were included, but still not much more variation could be explained, probably due to a smaller sample size than was the case with socioeconomic data. The total R2 values were 23,8% for actual gas use per m2 as dependent variable and 40,9% for DBTA (difference between the theoretical and actual consumption) per m2 as dependent variable. In both regression analyses, the majority of explanatory power for the DBTA came from dwelling characteristics. Household and occupant mattered less, although it was clear that the occupant behaviour data provided by the survey had a non-negligible predictive power for actual gas use per m2 of 9,1%. The fact that dwelling characteristics dominate the performance gap emphasises the importance of the assumptions made in the calculation method.
Besides the regression analyses for the total sample, the model was tested on under and for overpredictions separately, since the hypothesis was that these two phenomenon would be explained by different variables. There was a large difference in the amount of variation that could be explained by all available variables in these two samples. In the underpredicted set of data 19,9% of variation could be explained by occupancy presence patterns, presence of a programmable thermostat and water saving shower head. On the other hand, in overpredictions as much as 50,8% of variation was accounted for by dwelling and installation type, age of the building, floor area, and indoor temperature. Furthermore, reported comfort was a significant predictor only in overpredictions.
The results demonstrate the difficulty of finding the right predictors for actual
gas consumption. In the future both survey and socio demographic data could be combined to maximize the results, large samples should be used to ensure statistical significance and certain variables should probably be monitored in order to avoid survey bias. This includes variables like presence at home, indoor temperature an ventilation practices, since it seems that respondents might not be aware of their patterns well enough.
The relation between the performance gap and the normalised assumptions
Since the regression analyses did not cover the effect of variables such as indoor temperature, insulation quality, internal heat load etc. and this data was not available at that time, sensitivity of the theoretical calculation for certain parameters was conducted to fill this gap. Results showed, that an indoor temperature 2,7 degrees higher than assumed by the method currently (18 degrees) can explain the performance gap observed in label A and an indoor temperature 5,6 degrees lower than 18 degrees can account for the gap in label G. Both these temperature deviations are realistic, since people in well insulated dwellings probably heat their house more due to the small increment this causes in their monthly bill. Moreover, the installation system itself might be encouraging the occupants to heat more or less with for example low temperature floor heating installation in case of A labelled dwelling and with a local gas stove placed only in the living room in case of dwelling G. In the normalised calculation, all rooms are assumed to be heated. Heat resistance of the construction elements also had a big impact which demonstrates that in case of a poor inspection, the dwelling consumption could be very faulty due to an inaccurate estimation of insulation. This likely occurs in many old dwellings, where documentation is not available. Small increments in ventilation rates (up to 40% smaller or larger than current assumption) can also explain the performance gaps in label classes A to C. The two variables which had a smaller impact were the number of occupants and internal heat gains.
Longitudinal study confirmed the significant influence of insulation value by showing that the largest performance gaps appear in dwellings with poor envelope insulation, followed by those by poor window insulation. Considerable gaps appeared also in cases of heating installation of low efficiency.
A better model for theoretical gas consumption
Besides the exploratory regression analyses two other regression models were conducted in order to see whether the current theoretical consumption can be adapted with the new knowledge about the actual gas use. One model was made for under- and one for overpredicted consumptions. These models consisted of actual gas use as the dependent variable and theoretical gas consumption plus all other dwelling related features as predictors. The idea was to obtain the best possible theoretical consumption using only dwelling parameters so that the result could still be comparable among the dwellings. In the future, this could allow for determination of a more accurate dwelling consumption based only on dwelling parameters and average actual consumption data. For overpredictions, the model explained 33,8% of variation with installation and dwelling type being the significant variables (besides theoretical gas use). The explained variation was lower than for underpredictions, where it reached 60,0%, probably because the gap itself is much larger in overpredicted dwellings than in underpredictions.
The B coefficients obtained in these two models were then applied onto a different sample to see if a better predicted theoretical consumption could be obtained by adjusting the current theoretical use with the newly obtained parameters. The new theoretical consumption was indeed much closer to the actual gas use, which proves that this method could be used to obtain a better estimate of theoretical consumption.
Conclusion
There is a clear gap between actual and theoretical consumption in Dutch dwellings. Low performing dwellings tend to have a theoretical consumption much higher than actual, while high performing dwellings feature the opposite trend. These discrepancies are understandable at the level of individual dwellings and arise due to the standardizations made when calculating the theoretical consumption, however, on the level of the dwelling stock such a discrepancy is misleading and can lead to inaccurate policy reduction targets and sends wrong signals to several stakeholders (local governments, construction industry, renters and buyers etc.).
Regarding the causes of the discrepancies, they can party be explained by the features of the dwelling itself, meaning that the calculation model does not represent the reality accurately. However, a part of the discrepancy originates in the behaviour of the users and this part is difficult to quantify statistically. The results seem to indicate that underprediction is more difficult to explain and therefore probably more dependent on occupant practices than on the accuracy of the standardisation model. Overpredictions on the other hand, seem to have a lot in common with the fact that installation systems and the dwelling itself perform differently than expected. A methodological improvement seems to be more appropriate for the overpredicted cases while at the same time tackling the fact that occupants of these dwellings are likely to feel cold. For underpredictions on the other hand, changes to the methodology would mean accepting that a higher heating intensity is inevitable in efficient dwellings. While this should be further researched in the future, behaviour incentives that would encourage people to use their homes more wisely and not waste energy could be more successful.
The label calculation is easy to use and can be, as shown in the thesis, a very valuable tool for following the energy efficiency of the dwelling stock. Since the accuracy of theoretical gas and electricity calculations can easily be improved, it is a pity to miss the opportunity to do so. Several recommendations for further research and policy development were proposed regarding the methodology for the calculation of theoretical consumption. Examples of this are a revision of several standardised factors, revision of method for determining the insulation values on-site and introduction of correction factors based on actual consumption statistics. Moreover, labels that are issued should be accurate and reliable, meaning that more attention should be paid to the quality of inspections and the robustness of the software used for label calculation.
This thesis demonstrated that research on the relationship between policy instruments and their effects is crucial to ensure the effectiveness and a continuous improvement of these tools. Theoretical models, such as energy labelling, are often used to support policy decisions. As was shown, such models do not always provide results that correspond to reality, and in the case of dwellings a big reason for this is disregarding the user, who seems to adapt to the thermal quality of the house itself. However, as was demonstrated, there is a clear need for a more accurate estimation of consumption on a broader, dwelling stock level in order to enhance the effectiveness of the current renovation policies. moreover, showed that a better estimation is feasible. The thesis showed that using the current knowledge and data availability, there is few reason not to reduce the performance gap and predict the dwelling consumption more accurately.
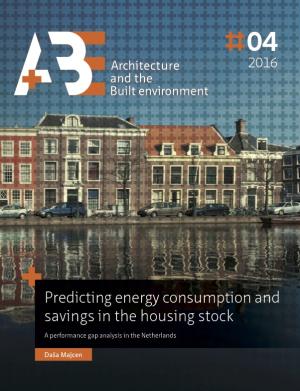